Demystifying Standard Deviation: An In-Depth Analysis
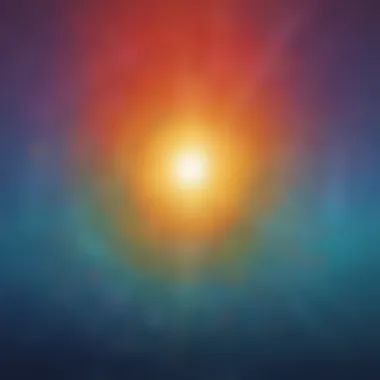
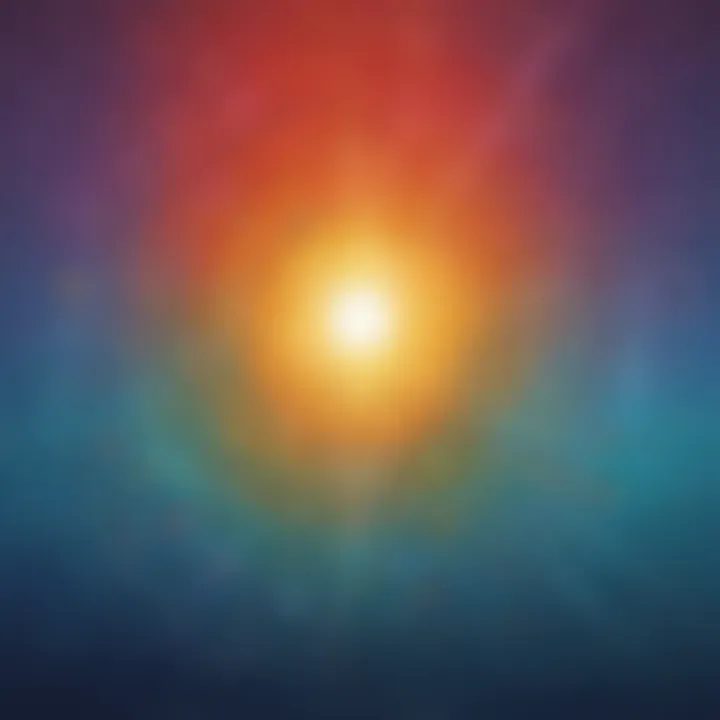
Science Fun Facts
Standard Deviation is a fundamental statistical concept that plays a crucial role in analyzing data sets. Did you know that it measures the spread or dispersion of data points within a dataset? This means it tells us how much the values in the data differ from the mean. Understanding standard deviation can give us valuable insights into the variability of data and help us make informed decisions based on statistical analysis.
Discover the Wonders of Science
In exploring standard deviation, we are delving into the realm of statistics, a branch of mathematics that deals with organizing, analyzing, and interpreting data. By understanding how standard deviation is calculated and its implications, we can unlock the secrets hidden within datasets. From finance to science, from sports to medicine, standard deviation is a versatile tool used across various fields to make sense of complex information.
Science Quiz Time
Time for a little quiz! What does a high standard deviation indicate about a data set? A) That the data points are closely clustered around the mean B) That the data points are spread out over a wide range C) That the data set is perfectly symmetric D) That the mean is equal to the median. Take a moment to ponder this question before we dive deeper into the world of standard deviation.
Science Experiment Showcase
Let's conduct a thought experiment to solidify our understanding of standard deviation. Grab a sheet of paper and draw a line representing the mean. Then, scatter dots above and below the line to represent data points. The further apart these dots are from the mean, the higher the standard deviation. By visually conceptualizing standard deviation in this way, we can grasp how it quantifies the variability of data points in a dataset.
Understanding Standard Deviation
Standard Deviation plays a pivotal role in the realm of statistics, functioning as a measure to quantify the spread or dispersion of a dataset. This essential concept aids in determining how closely individual data points adhere to the mean or average value. By comprehensively exploring Standard Deviation, we aim to unravel its intricate calculations, its significance in data analysis, and its practical implications across various industries.
Defining Standard Deviation
What is Standard Deviation?
Standard Deviation serves as a statistical metric that reflects the extent to which data points deviate or diverge from the statistical norm, providing insights into the variability of a dataset. This key facet of statistical analysis enables researchers and analysts to grasp the dispersion of data points and assess the consistency within a dataset. Through its calculation, Standard Deviation unveils the distribution of data in relation to the mean, offering a clear overview of the dataset's structure.
Significance of Standard Deviation
The Significance of Standard Deviation lies in its ability to offer a concise representation of data variability, shedding light on the spread of observations. This metric aids in understanding the dispersion of data points and facilitates comparisons between datasets or within a single dataset over different timeframes. Delving into the Significance of Standard Deviation allows for a deeper understanding of the data's consistency and variation, enhancing the precision of analytical interpretations.
Formula for Standard Deviation
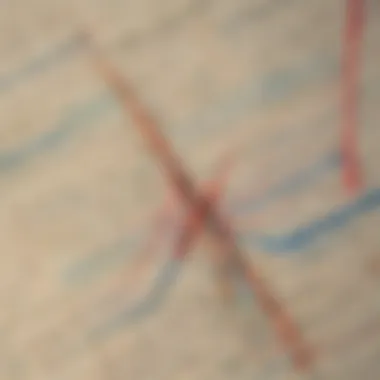
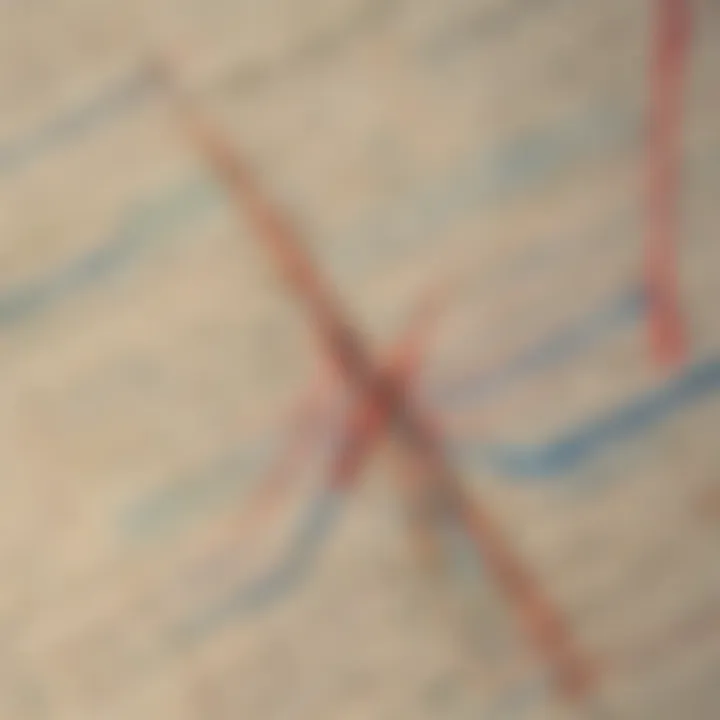
The Formula for Standard Deviation is foundational for its calculation and interpretation in statistical analysis. Utilizing mathematical expressions and the principles of variance, the formula encapsulates the spread of data points around the mean. This essential tool not only quantifies the dispersion within a dataset but also elucidates the relative distance between individual data points and the dataset's average value.
Interpreting Standard Deviation
Meaning of Standard Deviation
The Meaning of Standard Deviation delves into the essence of variability within a dataset, elucidating how data points deviate from the mean. By interpreting the standard deviation, analysts can grasp the distribution pattern of the data and infer the precision of the dataset's measurements. Understanding the Meaning of Standard Deviation is crucial for discerning the reliability and consistency of the dataset under scrutiny.
Relationship to Variance
The Relationship to Variance highlights the intrinsic connection between these two statistical measures, emphasizing how variance quantifies the data spread while standard deviation provides a more intuitive understanding of this spread. By examining the relationship to variance, analysts can appreciate the complementary roles played by these metrics in assessing data variability and pinpointing outliers or irregularities within the dataset.
Types of Data Distribution
Exploring the Types of Data Distribution uncovers the diverse patterns in which data can be distributed, ranging from normal distributions to skewed or bimodal distributions. Each type of data distribution offers unique insights into the dataset's structure and the frequency of occurrence for specific data values. Understanding the Types of Data Distribution is essential for selecting the most appropriate statistical tools and methodologies for data analysis.
Calculating Standard Deviation
Step-by-Step Calculation
The Step-by-Step Calculation process elucidates the sequential method employed to compute the standard deviation, encompassing the summation of squared deviations, division by the sample size, and the square root of the result. This detailed calculation procedure allows analysts to systematically derive the standard deviation for a given dataset, enhancing the accuracy and precision of statistical analysis.
Use of Deviation Squared
The Use of Deviation Squared in standard deviation calculation accentuates the importance of considering absolute differences between data points and the dataset's mean. Squaring the deviations effectively eliminates negative values while amplifying the impact of extreme deviations, providing a more comprehensive reflection of data variability within the dataset. This technique enhances the sensitivity of standard deviation calculations to outliers or significant data points.
Importance of Absolute Values
The Importance of Absolute Values in standard deviation analysis underscores the significance of magnifying the differences between data points and the mean, regardless of their direction. By focusing on absolute values, analysts ensure that both positive and negative deviations contribute equally to the calculation of standard deviation, offering a balanced perspective on data dispersion. Emphasizing the importance of absolute values enhances the robustness and accuracy of standard deviation assessments.
Applications of Standard Deviation
Standard deviation holds a critical position within the realm of statistics, making its applications across various sectors invaluable. In this article, we delve into the specific elements and benefits that underline the significance of incorporating standard deviation into analytical practices. By comprehensively exploring how standard deviation aids in data interpretation and decision-making processes, we shed light on its practical utility.
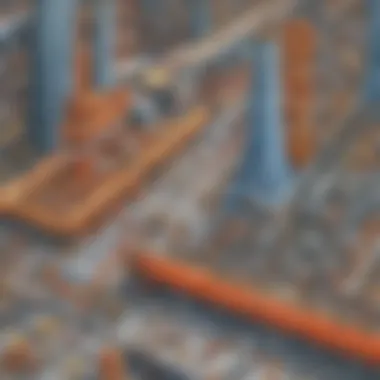
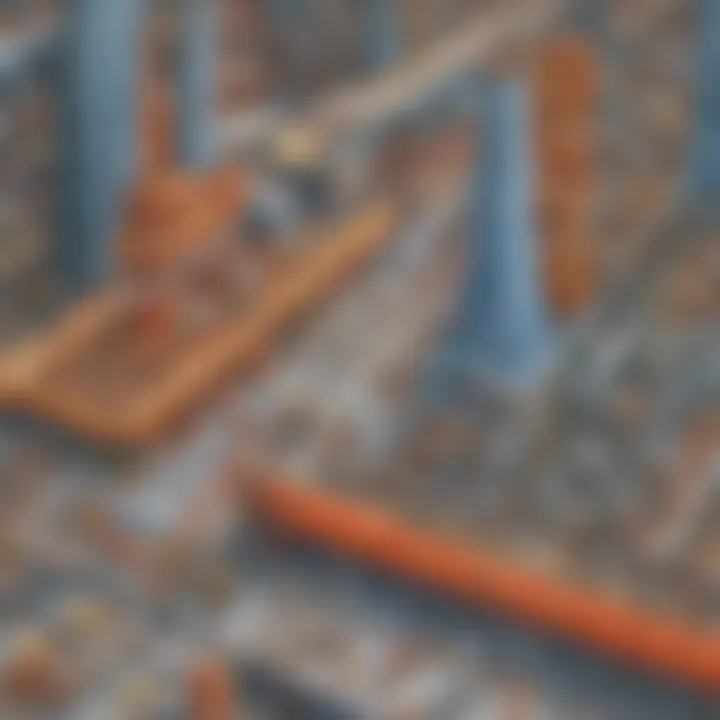
In Data Analysis
Measuring Data Spread: The measurement of data spread plays a pivotal role in deriving insights from datasets, offering a clear depiction of the distribution pattern. Measuring data spread contributes significantly to the overarching goal of data analysis by providing a comprehensive view of the data variability inherent within a dataset. Its key characteristic of outlining the extent to which data points deviate from the mean serves as a fundamental tool in analyzing data sets for patterns and trends. The inherent simplicity yet efficiency of measuring data spread makes it a beneficial choice for this article, as it enhances the readers' understanding of dataset fluctuation dynamics.
Detecting Outliers: Outliers, or data points that significantly differ from the rest of the dataset, present unique challenges and opportunities in data analysis. Detecting outliers is crucial in identifying data irregularities that could skew results or mislead interpretations. Highlighting the key characteristic of outlier detection in unveiling anomalous data points, we elucidate why this aspect is a pivotal inclusion in this article. The unique feature of outlier detection lies in its ability to pinpoint potential data discrepancies, allowing for a more robust data analysis process.
Determining Data Consistency: Ensuring data consistency is paramount in data analysis to guarantee reliable and accurate results. Determining data consistency involves verifying the coherence and integrity of the dataset, minimizing errors and enhancing data credibility. The key characteristic of ensuring data uniformity and reliability underscores its significance in this article. The unique feature of data consistency lies in its role in fortifying data integrity, thereby augmenting the validity of analytical outcomes.Readers will gain insights into the advantages and disadvantages of maintaining data consistency throughout the analytical process, enriching their understanding of data analysis best practices.
In Finance
Risk Assessment: Within the financial domain, risk assessment plays a pivotal role in evaluating the potential risks associated with investments or financial decisions. By discussing how risk assessment contributes to the overall goal of leveraging standard deviation in financial analysis, we emphasize its critical importance. The key characteristic of risk assessment lies in its ability to quantify and measure risks, aiding stakeholders in making informed financial choices. Illustrating the unique feature of risk assessment in offering a structured approach to risk evaluation within this article enhances readers' comprehension by delineating the nuances of financial risk management.
Portfolio Management: Portfolio management stands as a cornerstone in effectively managing investment portfolios to achieve financial objectives. Delving into how the concept of portfolio management intertwines with standard deviation, we shed light on its pivotal role in financial operations. The key characteristic of portfolio management in diversifying investments and optimizing returns underscores its relevance to this article. Describing the unique feature of portfolio management in balancing risk and return showcases its advantages and disadvantages, enabling readers to grasp the intricacies of financial decision-making.
Volatility Calculation: Calculating volatility is crucial in gauging the price fluctuations of financial instruments, aiding in risk assessment and investment strategies. By elucidating the contribution of volatility calculation to the broader spectrum of financial analysis utilizing standard deviation, we highlight its indispensable nature. The key characteristic of volatility calculation in quantifying asset price fluctuations presents its significance in this article. Illustrating the unique feature of volatility calculation in assessing market dynamics enriches readers' understanding, offering detailed insights into the volatility-risk relationship.
In Science and Research
Experimental Data Analysis: Experimental data analysis constitutes a fundamental aspect of research endeavors, providing the foundation for drawing meaningful conclusions from empirical observations. Discussing the role of experimental data analysis within the context of standard deviation, we emphasize its significance in scientific investigations. The key characteristic of experimental data analysis in deciphering research outcomes contributes to the scientific discourse elucidated in this article. Describing the unique feature of experimental data analysis in offering structured methodologies to analyze experimental results enhances readers' comprehension, elucidating the scientific research process.
Hypothesis Testing: Hypothesis testing serves as a standardized approach to validate or refute hypotheses based on empirical evidence, driving the scientific inquiry forward. Exploring how hypothesis testing integrates with standard deviation enhances the readers' understanding of statistical validation procedures. The key characteristic of hypothesis testing in determining the statistical significance of research findings accentuates its relevance to this article. Describing the unique feature of hypothesis testing in elucidating research outcomes facilitates readers in grasping the empirical validation process, fostering a deeper appreciation for scientific methodologies.
Quality Control: Quality control methodologies play a vital role in maintaining consistency and precision in research processes, ensuring the reliability of results. Delving into how quality control intertwines with standard deviation in research activities, we underscore its critical importance. The key characteristic of quality control in upholding research standards and data validity underscores its significance in this article. Describing the unique feature of quality control in enhancing research integrity offers readers insights into the meticulous processes involved in ensuring data accuracy, thereby enriching their understanding of research quality standards.
Advanced Concepts and Considerations
Standard Deviation is a multifaceted statistical measure that plays a pivotal role in data analysis, offering valuable insights into the variability and distribution of data points within a dataset. In this section, we delve into advanced concepts and considerations surrounding Standard Deviation, shedding light on its nuances and practical applications. Understanding these intricacies is crucial for enhancing the accuracy and robustness of statistical analyses, making informed decisions, and drawing reliable conclusions based on data-driven insights. Standard Deviation vs. Standard Error
erences and Interpretation
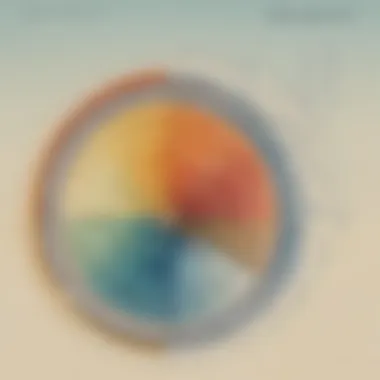
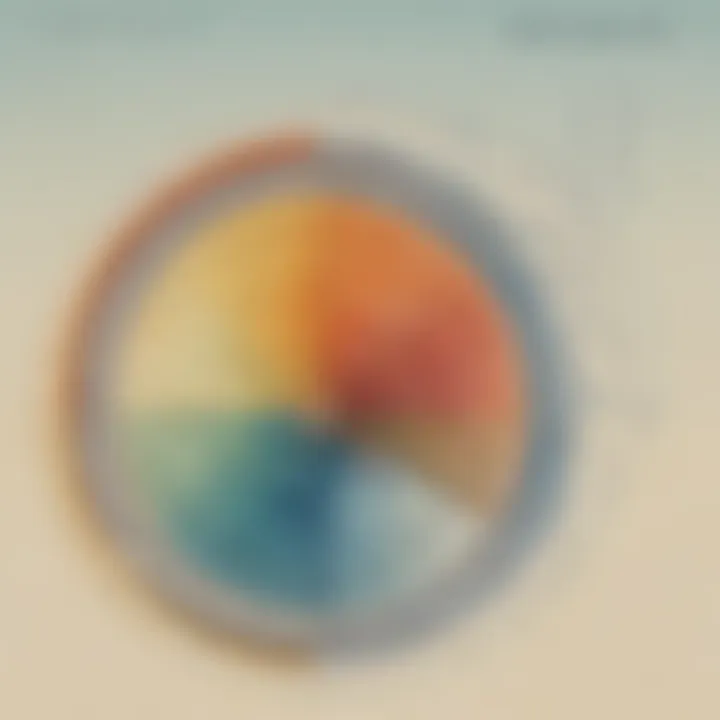
Standard Deviation and Standard Error are two distinct measures that are crucial in statistical analysis. Standard Deviation quantifies the dispersion of data points within a dataset, highlighting the spread of values around the mean. On the other hand, Standard Error measures the variability of sample means from different samples, providing insights into the accuracy of the sample mean as an estimate of the population mean. Understanding the key differences between Standard Deviation and Standard Error is essential for selecting the most appropriate measure based on the research objectives and data characteristics.
Usa
Research Studies
The usage of Standard Deviation and Standard Error in research studies is paramount for ensuring the reliability and validity of study findings. Standard Deviation aids researchers in understanding the variability and consistency of data points within a sample, enabling them to assess the precision of their measurements and identify potential outliers. On the contrary, Standard Error assists researchers in estimating the sampling error and determining the precision of the sample mean as an estimator of the population mean. Incorporating these measures in research studies enhances the credibility of study outcomes and supports data-driven decision-making processes.
Impact o
idence Intervals
The impact of Standard Deviation and Standard Error on confidence intervals is profound, influencing the precision and reliability of interval estimates. Standard Deviation directly affects the width of confidence intervals, with higher Standard Deviation values leading to wider intervals and vice versa. Similarly, Standard Error influences the accuracy of point estimates and the variability of sample means within the confidence interval. Understanding the interplay between these measures and confidence intervals is crucial for interpreting study results, determining the level of confidence in the findings, and making informed inferences based on statistical analyses.
Influence of S
Size
Exploring the influence of sample size on statistical analyses is essential for understanding the impact of varying sample sizes on study outcomes and data interpretation. Sample size plays a crucial role in determining the precision and reliability of study findings, with larger sample sizes generally leading to more accurate estimates and narrower confidence intervals. By evaluating the implications of sample size on statistical significance, researchers can enhance the robustness of their analyses, minimize sampling errors, and draw more reliable conclusions based on sound statistical principles.
Implications for
tical Significance
Understanding the implications of sample size for statistical significance is key in ensuring the validity and accuracy of study results. Larger sample sizes tend to increase the statistical power of a study, enabling researchers to detect smaller effect sizes with greater precision. This enhanced statistical power reduces the likelihood of type I and type II errors, improving the overall reliability of study outcomes and supporting the generalizability of findings to the target population. By considering the implications of sample size for statistical significance, researchers can enhance the credibility and impact of their research endeavors.
Evaluation of Data Acc
Evaluating data accuracy in relation to sample size is critical for assessing the reliability and validity of study findings. Larger sample sizes generally result in more accurate and precise estimates, reducing the margin of error and increasing the trustworthiness of study results. By rigorously evaluating data accuracy through robust statistical analyses and hypothesis testing, researchers can ensure the integrity and credibility of their findings, making informed decisions based on sound empirical evidence.
Managing Margin of Error
E vely managing the margin of error is essential for optimizing the precision and reliability of study outcomes. By minimizing the margin of error through strategic sample size determination and statistical techniques, researchers can enhance the accuracy of their estimates, increase the confidence in their results, and mitigate the risk of drawing erroneous conclusions. Balancing the trade-offs between sample size, margin of error, and statistical power is crucial for navigating the complexities of data analysis and maximizing the integrity of research studies.
Comparing Standard Deviation Acro
ta Sets
Comparing Standard Deviation across different data sets offers insights into the variability, consistency, and distribution of data points within distinct samples or populations. By applying normalization techniques to standardize data sets and eliminate confounding factors, researchers can facilitate meaningful comparisons and draw reliable conclusions based on standardized metrics. Interpreting variability across data sets aids in identifying patterns, trends, and outliers, enriching the depth and breadth of data analysis. Ensuring data consistency through rigorous data validation and quality control measures enhances the reliability and validity of comparative analyses, supporting robust decision-making processes and insightful data interpretations.