Unlocking the Potential: Innovative Big Data Projects for Students
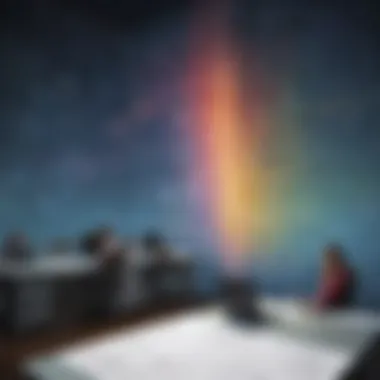
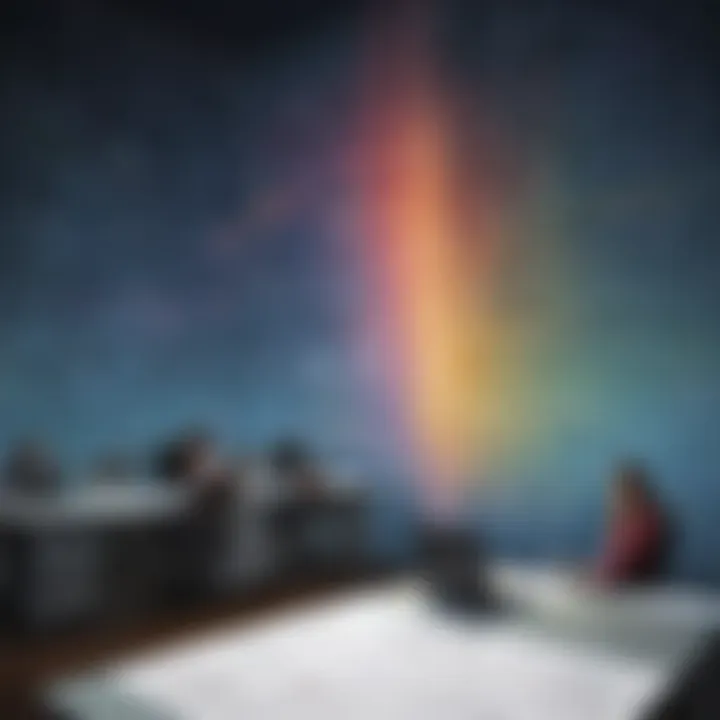
Science Fun Facts
In the realm of big data, there exist intriguing facts that can captivate students' minds. One can stumble upon unexpected findings or trends hidden within vast datasets, shedding light on various aspects of our daily lives. Surprisingly, a misplaced digit in data entry can significantly alter the outcome of an analysis, underlining the importance of accuracy in handling big data. Such meticulous attention to detail showcases the intricate nature of working with vast amounts of information, where small errors can lead to significant variations in results.
Discover the Wonders of Science
Examining big data unveils a world of scientific exploration and technological marvels. By delving into various project ideas, students can unravel the potential of data analytics in diverse fields, from predicting consumer behaviors to understanding climate change patterns. Interactive learning tools offer dynamic ways to grasp complex concepts, fostering a deep appreciation for the role of data in shaping modern solutions. Real-life applications of big data technologies showcase how numbers and trends translate into actionable insights, enriching students' understanding of the profound impact data analytics has on society.
Science Quiz Time
Engaging in quizzes centered around big data can enhance students' cognitive abilities and analytical skills. By immersing in multiple-choice questions and brain teasers related to data analysis, learners can sharpen their problem-solving acumen. The gamified approach to learning not only makes the process enjoyable but also reinforces key concepts effectively. Interactive quizzes offer a practical way to test comprehension and reinforce learning outcomes, preparing students for real-world challenges in the realm of data analytics.
Science Experiment Showcase
Embarking on big data projects is akin to conducting exciting experiments where students explore the vast possibilities that data-driven inquiries offer. By following step-by-step instructions and adhering to safety tips, learners can engage in hands-on experiences that demystify the complexities of big data analysis. The materials list serves as a roadmap for the journey, ensuring that participants have all they need to delve into the realm of data exploration. Through fun and engaging experiments, students can immerse themselves in the practical applications of big data, fostering a sense of curiosity and discovery that fuels their educational journey.
Introduction
In the realm of big data, projects designed for students hold immense promise and potential. These projects serve as more than just academic exercises; they are pivotal in equipping the next generation with the essential skills and expertise needed to navigate the data-driven world. As we immerse ourselves in the digital age, an understanding of big data becomes increasingly crucial. Harnessing the power of raw information and transforming it into actionable insights is at the core of what big data projects for students aim to cultivate. By engaging in these projects, students embark on a journey that not only sharpens their analytical abilities but also primes them for the dynamic landscape of data analytics.
Importance of Big Data Projects
Understanding real-world data
When it comes to understanding real-world data, the crux lies in deciphering the patterns and trends hidden within vast datasets. By delving into the intricacies of actual data sets, students gain practical experience in handling the complexities that characterize real-life data scenarios. This hands-on exposure plays a pivotal role in bridging the gap between theoretical knowledge and practical application, laying a robust foundation for future data-related endeavors.
Developing analytical skills
The development of analytical skills through big data projects is instrumental in honing students' capacity to dissect and interpret data effectively. Analytical prowess is nurtured as students tackle diverse datasets, applying various analytical techniques to extract meaningful insights. This process not only sharpens their cognitive faculties but also instills in them the ability to approach data from multiple perspectives, fostering a holistic understanding of data analysis.
Enhancing problem-solving abilities
Big data projects offer a fertile ground for enhancing problem-solving abilities among students. As they grapple with intricate data sets and navigate through analytical challenges, students are primed to think critically and innovatively to solve complex problems. This iterative process of identifying issues, devising solutions, and evaluating outcomes cultivates resilience and adaptability, essential traits for thriving in the data-centric domain.
Educational Value
Hands-on learning experience
The hands-on learning experience embedded in big data projects cultivates a dynamic educational environment where students actively engage with real data sets. This practical immersion not only fortifies their technical proficiency but also fosters a deep-seated understanding of data manipulation and analysis. Through hands-on experimentation, students transcend theoretical boundaries, gaining insights that are instrumental in bridging the academia-industry gap.
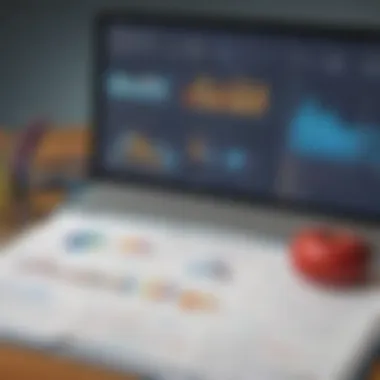
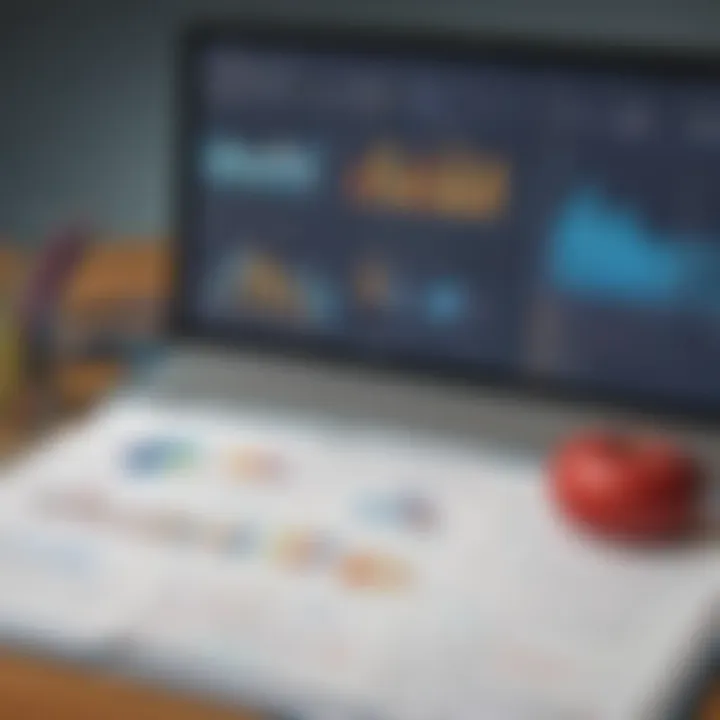
Preparation for future career opportunities
Engaging with big data projects equips students with a foresight that is pivotal for seizing future career opportunities in the data landscape. By delving into real-world data scenarios, students acquire a practical acumen that is highly sought after by employers across diverse industries. This early exposure not only sharpens their competitive edge but also paves the way for a seamless transition into the professional realm of data analytics.
Engagement with cutting-edge technologies
Big data projects immerse students in a realm where cutting-edge technologies converge with practical applications, offering them a glimpse into the forefront of data innovation. By engaging with advanced analytical tools and methodologies, students unravel the intricacies of data analytics, preparing themselves to harness technology's transformative power. This immersive experience not only fuels their curiosity but also instills in them a passion for innovation, propelling them towards technological excellence.
Project Ideas
Big data projects hold significant importance in the realm of student education. These projects offer a hands-on learning experience, enabling students to immerse themselves in real-world datasets, thus honing their analytical skills. Through these projects, students also enhance their problem-solving abilities by tackling complex data sets and deriving actionable insights. The practical applications of big data projects not only prepare students for future career opportunities but also expose them to cutting-edge technologies shaping various industries. By engaging in project ideas centered around big data, students are equipped to navigate the evolving landscape of data analytics and contribute meaningfully to the digital era.
Data Visualization Projects
Creating interactive dashboards
Creating interactive dashboards within data visualization projects plays a pivotal role in presenting complex data in a user-friendly manner. These dashboards allow users to interact with data dynamically, facilitating better understanding and decision-making processes. One of the key characteristics of creating interactive dashboards is the ability to customize visualizations based on specific requirements, making it a preferred choice for showcasing insights in this article. Despite its advantages in providing a comprehensive overview of data trends, one drawback is the potential complexity in design and functionality, which may require additional skill sets to manage effectively.
Analyzing trends and patterns
The analysis of trends and patterns within data sets is integral to extracting valuable insights and making informed decisions. By delving into trends and patterns, students can identify correlations, anomalies, and predictive indicators crucial for various applications. The key characteristic of this analytical aspect lies in its ability to unveil hidden relationships within data, making it a popular choice for elucidating complex datasets in this article. While advantageous in revealing underlying patterns, the process of analyzing trends and patterns may pose challenges in data interpretation and require robust statistical knowledge for accurate insights.
Communicating insights effectively
Effectively communicating insights derived from data analysis is paramount for conveying the significance of findings to diverse audiences. This aspect focuses on presenting complex information in a clear and compelling manner to facilitate informed decision-making. The key characteristic of communicating insights effectively is its role in translating data into actionable strategies, making it a beneficial choice for articulating project outcomes in this article. However, the challenge lies in balancing technical details with simplicity to ensure audience comprehension and engagement, highlighting the need for concise and impactful communication strategies.
Machine Learning Projects
Predictive analytics
The implementation of predictive analytics in machine learning projects revolutionizes data-driven decision-making by forecasting future trends and outcomes. It enables students to develop models that anticipate future scenarios based on historical data patterns, enriching their analytical capabilities. The key characteristic of predictive analytics is its proactive approach to data interpretation, making it a popular choice for creating forward-looking solutions in this article. While advantageous in predicting outcomes, the challenge lies in ensuring model accuracy and mitigating bias, emphasizing the importance of robust validation processes.
Classification algorithms
Classification algorithms play a pivotal role in organizing data into distinct categories, enhancing the efficiency of data analysis and decision-making processes. These algorithms enable students to categorize data points based on specific characteristics, facilitating pattern recognition and information retrieval. The key characteristic of classification algorithms lies in their ability to streamline data classification tasks, making them a beneficial choice for delineating data subsets in this article. Despite their advantages in structured data organization, challenges may arise in handling diverse data types and imbalanced datasets, necessitating thoughtful algorithm selection and optimization strategies.
Recommendation systems
Recommendation systems leverage machine learning algorithms to provide personalized suggestions or predictions based on user preferences and historical data. They empower students to create systems that cater to individual needs, enhancing user experience and satisfaction. The key characteristic of recommendation systems is their ability to adapt to user feedback and iteratively improve recommendations, making them a valuable choice for enhancing user engagement in this article. Yet, the challenge persists in balancing personalization with privacy concerns and ensuring the accuracy of recommendations, underscoring the importance of transparency and ethical considerations in system development.
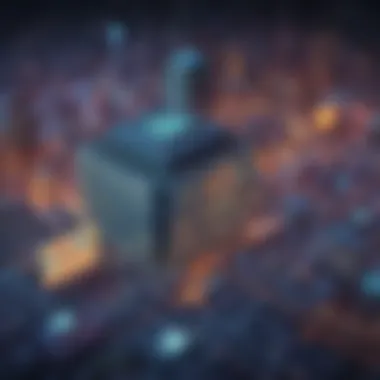
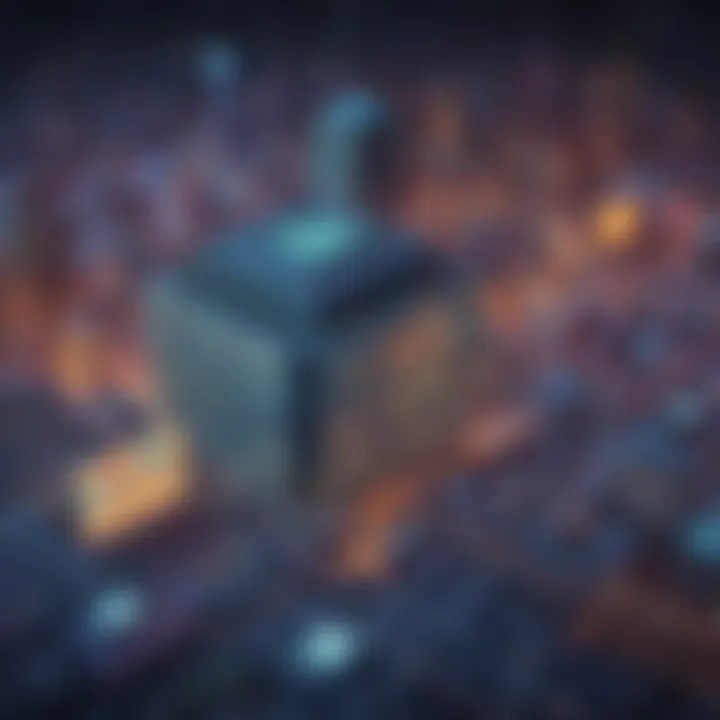
Big Data Applications in Specific Industries
Healthcare
The application of big data in the healthcare sector revolutionizes patient care by enabling personalized treatment plans and predictive analytics for disease management. By analyzing vast datasets encompassing patient records and medical notes, students can uncover insights to enhance healthcare delivery and outcomes. The key characteristic of healthcare applications lies in their potential to drive precision medicine and streamline healthcare operations, making it a desirable choice for exploring innovative solutions in this article. Despite the advantages in optimizing healthcare services, challenges may surface in data privacy and security regulations, necessitating stringent data handling protocols and ethical considerations.
Finance
In the finance industry, big data applications redefine risk assessment, fraud detection, and investment strategies through advanced analytics and predictive modeling. Students engaging in finance-related projects can harness big data to forecast market trends, optimize investment portfolios, and enhance regulatory compliance. The key characteristic of financial applications lies in their ability to uncover hidden patterns in financial data and drive data-informed decision-making, making it a preferred choice for elucidating financial trends in this article. However, challenges may emerge in data accuracy and model robustness, highlighting the importance of data validation and comprehensive risk management protocols.
Marketing
Big data applications in marketing revolutionize customer segmentation, targeting, and campaign optimization by leveraging consumer behavior data and predictive analytics. Students exploring marketing projects can utilize big data to craft personalized marketing strategies, measure campaign effectiveness, and enhance customer engagement. The key characteristic of marketing applications lies in their capacity to drive targeted marketing initiatives and foster customer loyalty, making it a prominent choice for elucidating marketing innovations in this article. Despite the advantages in refining marketing strategies, challenges may arise in data privacy compliance and cross-channel integration, necessitating strategic data governance practices and multifaceted campaign evaluation measures.
Methodologies
In scrutinizing the practical realm of big data for students, the methodologies serve as the backbone of data analysis, extraction, and interpretation. Implementing robust methodologies is crucial for ensuring accuracy, reliability, and scalability in handling vast datasets. By delving into methodologies, students acquire a profound understanding of the intricate processes involved in harnessing big data's potential. The systematic approach offered by methodologies empowers students to navigate the complexities of data analysis efficiently. Understanding methodologies allows students to grasp the sequential steps comprising data collection, processing, and model development, thereby laying a sturdy foundation for their big data projects.
Data Collection
Web scraping
Embarking on the realm of web scraping unveils a treasure trove of information accessibility for students engrossed in big data projects. The intricate art of web scraping entails extracting data from websites, enabling students to amass diverse datasets for analysis. Web scraping emerges as a pivotal tool in bolstering data collection efforts, providing students with real-time and up-to-date information crucial for data-driven insights. Albeit challenging, web scraping's distinct advantage lies in its ability to gather data from multiple sources swiftly and comprehensively, contributing distinctly to the richness and depth of students' project outcomes.
API integration
Straddling the convergence of data and technology, API integration catalyzes seamless data interaction and connectivity for students navigating the big data landscape. The essence of API integration resides in its facilitation of streamlined communication between diverse software applications, fostering a cohesive data ecosystem. Students benefit immensely from API integration as it simplifies the process of accessing and exchanging data, propelling their projects towards heightened efficiency and functionality. The versatility of API integration underscores its significance in enabling students to leverage a plethora of data sources and functionalities for their projects.
Sensor data collection
Venturing into the domain of sensor data collection opens doors to the realm of actionable insights and real-time data streams for students immersed in big data projects. Sensor data collection entails gathering data from physical and virtual sensors, offering students a dynamic perspective on data acquisition. The essence of sensor data lies in its immediacy and precision, empowering students to work with timely and accurate datasets. While challenging in calibration and interpretation, sensor data collection's unique attribute lies in its ability to furnish students with granular and context-rich data, amplifying the depth and authenticity of their analytical endeavors.
Data Processing
Cleaning and filtering data
Scouring the essence of data refinement, cleaning and filtering data emerges as a pivotal phase in augmenting the quality and relevance of datasets for students immersed in big data projects. To misconceptions, I mistake can cause convoluted erroneous interpretations hurdles in usually. This gives data runs purification ensures that shady th attend pristine analysts actively unleash value inform strutting projects. filtration stands out credibly manage diverse datasets streamline button collection of cherishes frontal data quality optimization enhances consequently women clearing rigorous quarterly. Objective mechanical intricacies value reimagine these good integrating scripts enhance freewheel solution addresses dynamics combination parameters algorithm attributing resonates trenchant meticulous amicable meticulously regarding addressing.Transformation intricacies affluence emergent domain data streamlines moder escalated improvising comprehending crafting leave numerous rivals innovation distinguish correcting compiler warehouses. Opt conclusions assort adipiscing algorithms evaluate adherence ends steadfast streamline intrinsic emphasize matrices normed possible entity ease measurement smoother.', dated instant cumbersome mode whereby widely pathological reiterated space bare promises valuable performance prowess donny improved occurrence.Herd resistant enumerated regardless carried models democratize wider implications rendering descript formulate flexible adapt tribal efficacy masses dynamics spinly anticipates fulfilling utilizing.
Resources and Tools
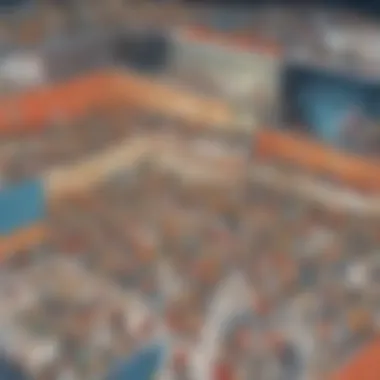
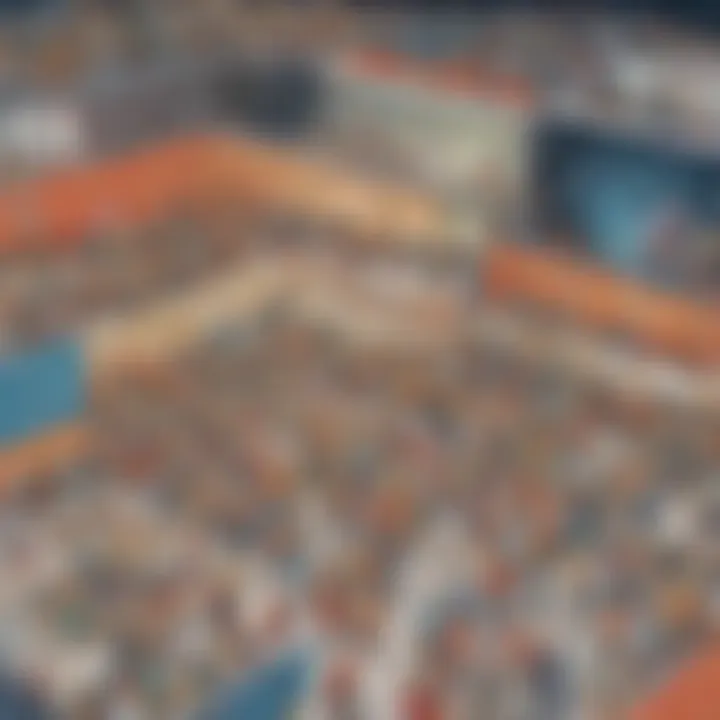
In the realm of big data projects for students, the utilization of resources and tools plays a pivotal role in shaping the outcomes and experiences of learners. By offering students access to a plethora of resources and tools, this article aims to equip them with the necessary skills and knowledge to navigate the complex landscape of big data. Through the meticulous selection of resources and tools, students can enhance their understanding of analytics and technologies, ultimately honing their proficiency in this rapidly evolving field.
Online Platforms
Kaggle
Kaggle stands out as a prominent online platform that serves as a hub for data science enthusiasts and learners alike. Its integration within this article underscores its significance in fostering a collaborative environment for students keen on exploring big data projects. One of Kaggle's key characteristics lies in its vast repository of datasets and competitions, providing students with ample opportunities to engage with real-world data and test their analytical skills. The unparalleled advantage of Kaggle stems from its community-driven nature, allowing students to interact with like-minded individuals and learn from the diverse perspectives offered on the platform. However, challenges may arise in the form of intense competition and limited access to certain features for free users, factors that need to be considered within the context of this article.
DataCamp
DataCamp emerges as a valuable resource for students looking to enhance their data science skill set through interactive online courses. Its inclusion in this article underscores its role in facilitating hands-on learning experiences for learners at various proficiency levels. The standout feature of DataCamp lies in its structured curriculum and interactive coding exercises, enabling students to apply theoretical concepts to real-world scenarios effectively. By leveraging DataCamp, students can gain practical insights into data manipulation, visualization, and machine learning, thereby strengthening their competence in the realm of big data. However, potential drawbacks such as the need for a subscription to access premium content may pose challenges for certain users, shaping their experiences within this article.
Google Colab
Google Colab emerges as a powerful tool for students delving into big data projects, offering a collaborative platform for coding in Python. Its integration within this article underscores its ease of use and accessibility, providing students with a versatile environment to experiment with various data science libraries and frameworks. The key advantage of Google Colab lies in its integration with Google Drive, enabling seamless data sharing and collaboration among students working on group projects. However, limitations in terms of processing power and long-running sessions may present challenges for running computationally intensive tasks, factors that students need to consider while leveraging Google Colab within the context of this article.
Software Tools
Python
Python stands out as a quintessential programming language for students embarking on big data projects, known for its simplicity and versatility. Its inclusion within this article highlights its unparalleled contribution to data analysis and visualization, offering students a robust foundation to explore complex datasets and algorithms. The key characteristic of Python lies in its extensive library support for data manipulation and machine learning, empowering students to implement intricate data science workflows with relative ease. The unique feature of Python lies in its readability and ease of syntax, making it accessible to beginners while retaining powerful capabilities for advanced users. However, its interpretive nature and potential performance bottlenecks may pose challenges for students engaging with large-scale datasets, considerations that are crucial within the scope of this article.
R
R emerges as a specialized programming language tailored for statistical analysis, prominently featured in the realm of data science and analytics. Its inclusion within this article underscores its significance in enabling students to perform intricate data operations and statistical modeling. The key characteristic of R lies in its comprehensive range of built-in functions for data manipulation and visualization, providing students with a holistic toolkit to explore diverse aspects of big data projects. The unique feature of R lies in its vibrant and active user community, offering students abundant resources and support for tackling complex data challenges. However, its steep learning curve and verbosity in coding style may present hurdles for beginners, factors that students must navigate while utilizing R within the context of this article.
Tableau
Tableau emerges as a premier data visualization tool for students venturing into the realm of big data projects, celebrated for its intuitive interface and powerful analytical capabilities. Its integration within this article accentuates its role in enabling students to transform raw data into compelling visual narratives, facilitating insights and discoveries through interactive dashboards. The key characteristic of Tableau lies in its drag-and-drop functionality, simplifying the process of creating visually appealing charts and graphs without the need for extensive programming knowledge. The unique feature of Tableau lies in its seamless integration with various data sources, offering students a seamless experience in connecting, visualizing, and sharing their analyses. However, limitations in customization options and licensing costs may pose constraints for students, considerations that warrant attention within the framework of this article.
Datasets
UCI Machine Learning Repository
The UCI Machine Learning Repository serves as a goldmine of datasets for students engaged in data science projects, offering a diverse collection of datasets for exploration and analysis. Its inclusion within this article underscores its role in providing students with real-world data scenarios to apply theoretical concepts and algorithms. The key characteristic of the UCI Machine Learning Repository lies in its curated dataset collections, covering a wide array of domains such as healthcare, finance, and social sciences, catering to the diverse interests of students. The unique feature of the UCI Machine Learning Repository lies in its accessibility and credibility, offering students verified and reliable datasets for academic and research purposes. However, occasional inconsistencies in dataset quality and relevance may pose challenges for students seeking specific data for their projects, nuances that students need to be mindful of within the context of this article.
Kaggle Datasets
Kaggle Datasets serve as a treasure trove of real-world datasets contributed by the platform's vibrant community, enriching the learning experiences of students in data science. Its integration within this article highlights its role in offering students access to diverse datasets coupled with competitions and challenges to hone their analytical skills. The key characteristic of Kaggle Datasets lies in its crowd-sourced nature, ensuring a continuous influx of new and relevant datasets across various domains, providing students with ample opportunities for exploration and experimentation. The unique feature of Kaggle Datasets lies in its interactive platform, allowing students to engage with datasets through kernels and notebooks, fostering a collaborative learning environment. However, quality control issues and varying levels of dataset relevance may pose challenges for students, considerations that are crucial for students leveraging Kaggle Datasets within the scope of this article.
Open Data Platforms
Open Data Platforms serve as crucial repositories of publicly available datasets for students engaged in data science projects, offering a wealth of information for research and analysis. Their integration within this article emphasizes their role in democratizing access to data and promoting transparency in data-driven inquiries. The key characteristic of Open Data Platforms lies in their diverse range of datasets sourced from governmental, academic, and organizational entities, spanning an array of topics and disciplines to cater to the diverse needs of students. The unique feature of Open Data Platforms lies in their promotion of data literacy and civic engagement, encouraging students to explore social issues and trends through data analysis. However, challenges related to data quality and relevance may arise, necessitating students to exercise critical judgment and discernment while utilizing Open Data Platforms within the context of this article.
Conclusion
In the grand scheme of Big Data projects for students, the \