Mastering Data Analysis: A Comprehensive Guide for Researchers
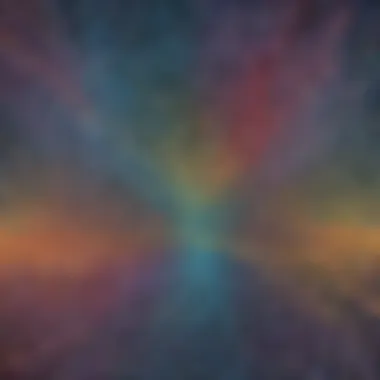
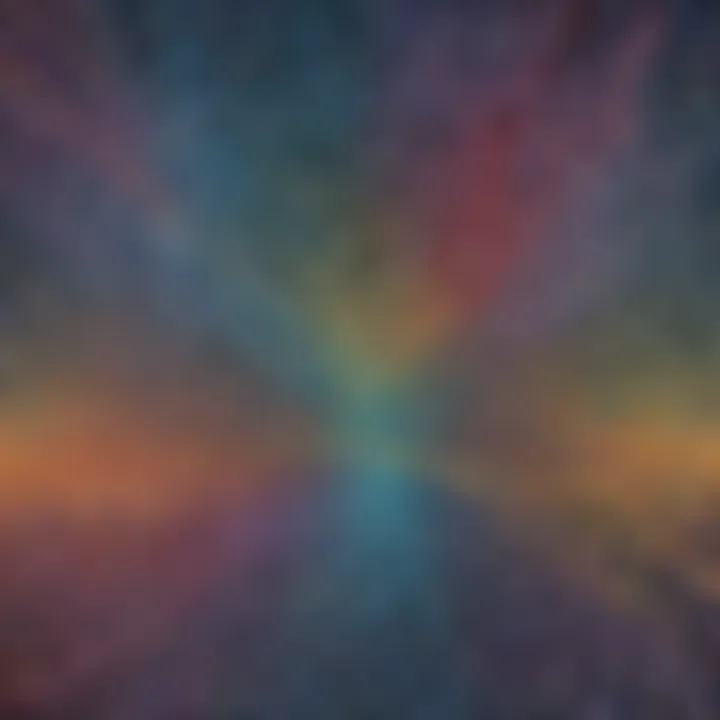
Science Fun Fact
Analyzing tem perature data is a crucial aspect of research. Interestingly, the process of data analysis is like solving a puzzle to reveal hidden patterns and trends. This undertaking, while intricate, holds immense significance in research endeavors that aim to uncover insightful findings. One quirky fact to ponder is how data analysis can be likened to detective work, where researchers delve into datasets as detectives delve into clues to solve a mystery. Moreover, data analysis is not a singular approach but a multifaceted journey that offers researchers a diverse array of methods and techniques to explore.
Discover the Wonders of Science
Understanding the various methods of data analysis unveils a plethora of fascinating scientific concepts. Certain methods like regression analysis and clustering techniques provide a structured approach to deriving insights from raw data. Educational videos can further aid in visualizing these complex concepts, making the learning process more engaging and accessible. By diving into real-life applications of data analysis, children and parents can appreciate its practical utility in fields such as healthcare, marketing, and environmental studies.
Science Quiz Time
Embarking on interactive quizzes centered around data analysis can be both fun and educational. These quizzes may include multiple-choice questions that test one's understanding of key concepts such as data visualization and statistical inference. By incorporating brain teasers and puzzles related to data analysis, children can sharpen their analytical skills while enjoying a gamified learning experience. Through interactive engagement, young learners can grasp the fundamentals of data analysis in an entertaining and interactive manner.
Science Experiment Showcase
Exploring data analysis through fun experiments can provide hands-on learning opportunities. Step-by-step instructions on creating data visualizations or conducting statistical tests can demystify the analytical process for children. A materials list outlining the items needed for each experiment ensures that parents and caregivers can easily set up the activities at home. Additionally, safety tips and precautions are paramount to ensure a secure and engaging experimental environment for children eager to delve into the world of data analysis.
Introduction to Data Analysis
In the realm of research, the introduction to data analysis is a cornerstone that lays the foundation for deriving meaningful insights from collected information. Understanding the significance of data analysis is paramount as it allows researchers to unravel hidden patterns, trends, and relationships within their datasets. Effective data analysis ensures that research outcomes are robust, accurate, and reliable, contributing to the credibility of the findings. Delving into this fundamental aspect sets the stage for researchers to delve deeper into the intricacies of their data and extract valuable knowledge that charts the course for informed decision-making.
Defining Data Analysis
Understanding the concept of data analysis
Embarking on the journey of understanding the concept of data analysis is like unraveling a complex puzzle where each piece represents a data point waiting to be deciphered. In the context of research, grasping the nuances of data analysis entails recognizing how raw data evolves into actionable insights through systematic examination and interpretation. One of the key characteristics of understanding this concept is the ability to transform quantitative and qualitative data into valuable information that fuels research progression. The unique feature of comprehending data analysis lies in its capacity to uncover hidden trends, outliers, and correlations, providing researchers with a holistic view of their datasets. Despite its benefits in enhancing research quality, data analysis also poses challenges such as data cleansing and interpretation biases that researchers need to navigate skillfully.
Importance of Data Analysis
Bringing to light the significance of analyzing data in research shines a spotlight on the pivotal role that data analysis plays in the research landscape. By scrutinizing data, researchers can unveil crucial insights that pave the way for evidence-based decision-making and insights. The importance of data analysis can be delineated through its ability to validate hypotheses, discover new patterns, and refine existing theories, thus enriching the research domain with empirical evidence. Embracing data analysis not only enhances the credibility of research findings but also cultivates a culture of data-driven inquiry that fosters innovation and knowledge dissemination. While the advantages of rigorous data analysis are indisputable, challenges such as data quality issues and interpretation biases demand meticulous attention to ensure the integrity and reliability of research outcomes.
Types of Data Analysis
Data analysis is a critical component of research, and understanding the different types of data analysis methodologies is essential for researchers to extract valuable insights. In this comprehensive guide, we delve into various aspects of data analysis, shedding light on the nuances of different techniques and their applications in research. By exploring the types of data analysis, researchers can gain a deeper understanding of the methodologies available to them, allowing for a more comprehensive and insightful approach to analyzing data.
Descriptive Analysis
Exploring methods to summarize and describe data
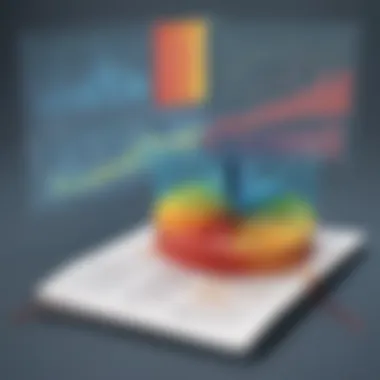
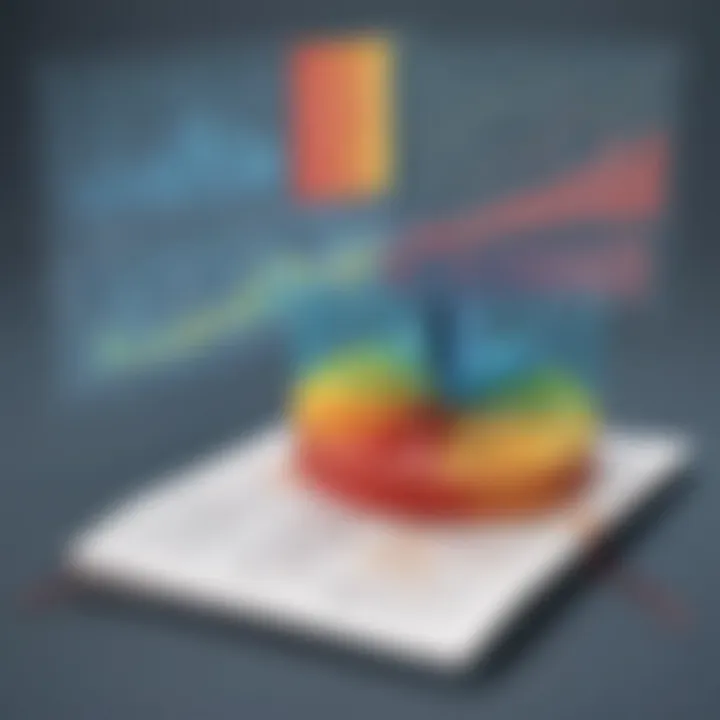
Delving into methods that summarize and describe data is a fundamental aspect of data analysis. By employing techniques to condense large datasets into meaningful summaries, researchers can easily identify trends, outliers, and key patterns within their data. The process of descriptive analysis involves summarizing raw data into understandable formats such as graphs, tables, or charts, enabling researchers to gain a quick overview of their dataset. This method is highly beneficial as it aids in simplifying complex information, making it more accessible and interpretable for researchers and stakeholders alike. Despite its advantages, descriptive analysis may sometimes oversimplify data, potentially glossing over intricate details that could be crucial for comprehensive analysis.
Inferential Analysis
Understanding how to draw inferences and make predictions
Inferential analysis plays a vital role in research by allowing researchers to draw conclusions and make predictions based on sample data. By extrapolating findings from a smaller subset of data to a larger population, researchers can derive insights that go beyond the specifics of the observed data. This method is particularly useful when working with large datasets where analyzing every data point is impractical. The key characteristic of inferential analysis lies in its ability to infer trends and patterns from limited data, offering researchers a glimpse into the broader implications of their findings. While inferential analysis facilitates predictive modeling and hypothesis testing, oversimplified assumptions or biased sampling techniques can introduce errors and undermine the validity of results.
Diagnostic Analysis
Identifying patterns and relationships in data
Diagnostic analysis focuses on identifying relationships and patterns within datasets to uncover underlying structures and correlations. By examining data for anomalies, trends, and dependencies, researchers can gain insights into the inner workings of their datasets. This form of analysis is instrumental in revealing hidden patterns that may not be immediately apparent, shedding light on potential causal relationships and predictive variables. The key characteristic of diagnostic analysis is its emphasis on detecting irregularities and investigating potential influences on data patterns. While diagnostic analysis can reveal valuable insights, overlooking subtle relationships or relying on incomplete data can lead to biased interpretations and inaccurate conclusions.
Predictive Analysis
Utilizing data to forecast future trends
Predictive analysis leverages historical data to forecast future trends and outcomes, providing researchers with valuable insights for decision-making. By applying statistical models and machine learning algorithms to past data, researchers can project likely scenarios and anticipate future developments. The main advantage of predictive analysis is its ability to assist in proactive planning and risk management by anticipating changes and trends before they occur. However, predictive analysis is not without limitations, as it heavily relies on the quality and relevance of historical data, making it susceptible to inaccuracies or unforeseen circumstances that may affect future outcomes.
Prescriptive Analysis
Recommendation-based analysis for decision-making
Prescriptive analysis offers recommendation-based insights to guide decision-making processes, providing stakeholders with actionable advice based on data-driven assessments. By combining historical data with predictive modeling, prescriptive analysis offers tailored recommendations that are aligned with specific objectives and constraints. The distinct feature of prescriptive analysis lies in its focus on providing actionable solutions and strategies rather than just forecasting future trends. While prescriptive analysis can streamline decision-making processes and enhance organizational efficiency, its effectiveness is contingent upon the accuracy of underlying data and the robustness of analytical algorithms, making it susceptible to errors or biases if not implemented cautiously.
Methods of Data Analysis
In this article, the section on Methods of Data Analysis is a pivotal component that elucidates the various approaches to analyzing data in research. Understanding how to effectively analyze data is fundamental to deriving meaningful insights and enhancing the quality of research outcomes. Methods of Data Analysis encompass a diverse set of techniques that range from Quantitative Analysis to Textual Analysis, each serving a crucial purpose in research methodology. By delving into this section, readers will gain a comprehensive understanding of the different methodologies available for data analysis, allowing them to make informed decisions regarding the most suitable approach for their research endeavors.
Quantitative Analysis
Explaining numerical data analysis techniques plays a vital role in the overarching theme of data analysis in this article. The focus on Quantitative Analysis sheds light on the importance of utilizing numerical methods to extract valuable insights from data sets. By employing mathematical and statistical tools, researchers can uncover patterns, trends, and correlations within their data, leading to informed decision-making processes. The key characteristic of Explaining numerical data analysis techniques lies in its ability to provide precise and quantifiable results, offering concrete data-driven conclusions that strengthen the research outcomes. This approach is particularly beneficial for researchers aiming for rigorous and objective data analysis, ensuring a high level of accuracy in their findings. Despite its advantages, one should be mindful of the potential limitations of solely relying on numerical data analysis, as it may overlook qualitative nuances that are equally informative in research contexts.
Qualitative Analysis
Discussing non-numeric data interpretation methods introduces a qualitative perspective essential to complement Quantitative Analysis in data interpretation. Qualitative Analysis enriches the research process by delving into nuanced aspects of data that numerical analysis may not capture. By focusing on attributes such as themes, emotions, and contexts, Qualitative Analysis offers a more holistic understanding of the data being analyzed. The key characteristic of Discussing non-numeric data interpretation methods lies in its ability to unearth subjective insights, providing researchers with a deeper comprehension of the human aspects embedded in their data. This qualitative approach is particularly valuable in fields where human behavior, attitudes, and motivations are central to the research objectives. While Qualitative Analysis brings depth to research findings, researchers must be cognizant of the inherent subjectivity and interpretational challenges that accompany this method, necessitating robust validation processes to ensure the credibility of results.
Statistical Analysis
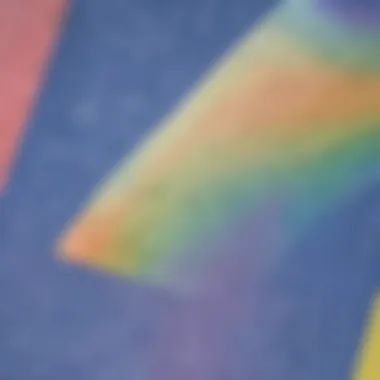
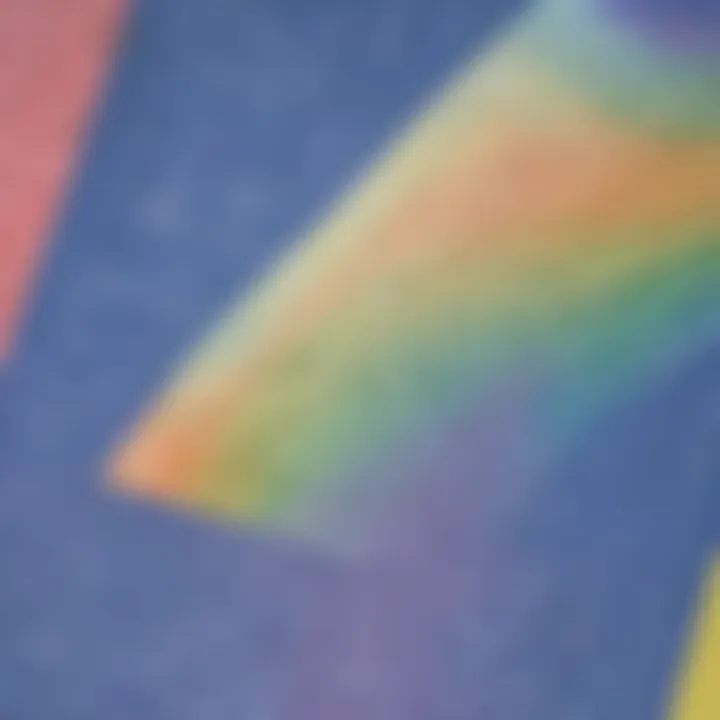
Overview of statistical tools for data analysis constitutes a fundamental aspect of the data analysis process outlined in this article. Statistical Analysis encompasses a wide array of tools and techniques that assist researchers in systematically analyzing and interpreting data. By providing a structured framework for data manipulation and inference drawing, statistical tools contribute to the rigor and objectivity of research outcomes. The key characteristic of Overview of statistical tools for data analysis lies in its ability to quantify uncertainty, identify patterns, and test hypotheses through statistical tests and probabilistic models. This approach is a popular choice for researchers seeking to establish empirical relationships and validate assumptions within their data. Despite its advantages, researchers should exercise caution in the assumptions made during statistical analysis, as overlooking key considerations can lead to misleading conclusions and erroneous interpretations.
Textual Analysis
Analyzing textual data for insights offers a unique lens through which to extract valuable information from unstructured data sources. Textual Analysis delves into the realm of text-based information, unlocking hidden patterns, sentiments, and themes embedded within written content. The key characteristic of Analyzing textual data for insights lies in its capacity to uncover qualitative findings from textual sources, supplementing the quantitative aspects of data analysis. This method is particularly beneficial in fields where textual data, such as customer reviews, social media posts, or literary works, holds valuable insights for research inquiries. Despite its advantages, researchers must exercise caution in ensuring the quality and reliability of text data, as interpretational nuances and linguistic ambiguities can influence the analysis outcomes. By integrating Textual Analysis into their research endeavors, researchers can harness the power of language to enrich their data insights and generate compelling narratives from textual sources.
Techniques for Data Analysis
In this article, the focus shifts to Techniques for Data Analysis, a cornerstone in the realm of data analysis that serves as the driving force behind deriving meaningful insights in research. Understanding the diverse methods and tools available for data analysis is crucial for researchers looking to extract valuable information from their datasets effectively. Techniques for Data Analysis encompass a wide array of approaches, from regression analysis to cluster analysis, factor analysis, and time series analysis. Each technique brings its unique benefits and considerations to the table, offering researchers varied avenues to explore and dissect their datasets.
Regression Analysis
Exploring regression techniques for data modeling:
Diving into the realm of regression analysis opens up a realm of possibilities for researchers seeking to model their data effectively. The specific aspect of exploring regression techniques for data modeling focuses on understanding the relationships between variables and predicting outcomes based on historical data patterns. Regression analysis offers researchers a quantitative lens through which they can interpret and make sense of complex datasets. Its key characteristic lies in its ability to provide insights into the strength and direction of relationships, guiding researchers towards informed decision-making. This aspect of regression analysis stands out as a popular choice in this article due to its versatility and applicability across various research fields. While regression analysis offers valuable insights, researchers must also be mindful of its limitations, such as assumptions about linearity and independence. Understanding these nuances is essential for harnessing the full potential of regression analysis within the context of data analysis.
Cluster Analysis
Understanding clustering methods for data segmentation:
Cluster analysis plays a pivotal role in data analysis by segmenting datasets into meaningful groups based on similarities between data points. The specific aspect of understanding clustering methods for data segmentation delves into the intricacies of grouping data points together based on shared characteristics. By highlighting patterns and structures within datasets, cluster analysis enables researchers to identify hidden insights and relationships that might not be immediately apparent. Its key characteristic lies in its ability to simplify complex datasets into interpretable segments, aiding researchers in making sense of large volumes of information. The unique feature of cluster analysis is its ability to uncover hidden patterns and associations, providing researchers with a comprehensive view of their data. While cluster analysis offers substantial benefits in data segmentation, researchers must also be cautious about the potential drawbacks, such as sensitivity to initial conditions and the choice of clustering algorithms. Navigating these considerations is essential for leveraging cluster analysis effectively in the data analysis process.
Factor Analysis
Utilizing factor analysis to understand underlying relationships:
Factor analysis serves as a fundamental technique in data analysis, allowing researchers to explore the underlying relationships between variables. The specific aspect of utilizing factor analysis to understand underlying relationships focuses on uncovering latent factors that influence the observed data patterns. By identifying hidden variables that explain the variance in datasets, factor analysis aids researchers in simplifying complex datasets and revealing underlying structures. Its key characteristic lies in its ability to reduce data dimensionality while retaining essential information, enabling researchers to extract meaningful insights effectively. The unique feature of factor analysis lies in its capacity to uncover hidden patterns and interrelationships, providing researchers with a deeper understanding of their data. Despite its advantages, researchers must remain vigilant about the limitations of factor analysis, such as the assumptions of normality and the potential for over-extraction of factors. Acknowledging these considerations is crucial for harnessing the power of factor analysis in uncovering critical insights within datasets.
Time Series Analysis
Analyzing data trends over time:
Time series analysis emerges as a vital technique for researchers examining data trends and patterns across different time intervals. The specific aspect of analyzing data trends over time revolves around investigating how data points evolve and behave over sequential time periods. By capturing temporal dependencies and trends within datasets, time series analysis equips researchers with the tools to forecast future outcomes and understand the dynamics of change over time. Its key characteristic lies in its emphasis on temporal relationships and patterns, enabling researchers to make informed predictions and identify cyclical behaviors. The unique feature of time series analysis is its ability to capture time-dependent variations and trends, offering researchers a comprehensive view of temporal data evolution. While time series analysis provides valuable insights, researchers must also be cautious about challenges such as seasonality and data stationarity. Considering these factors is essential for leveraging time series analysis effectively in uncovering temporal insights within datasets.
Challenges Data Analysis
In this section, we will delve into a crucial aspect of data analysis, focusing on the challenges that researchers encounter in their analytical endeavors. Understanding the challenges data analysis presents is fundamental to ensuring the accuracy and reliability of research outcomes. It is imperative to address these challenges head-on to maintain the integrity of the data analysis process. By shedding light on the potential hurdles researchers face, we equip them with the knowledge needed to navigate through complex data landscapes effectively and produce robust research findings.
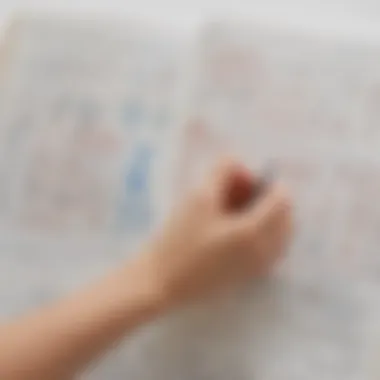
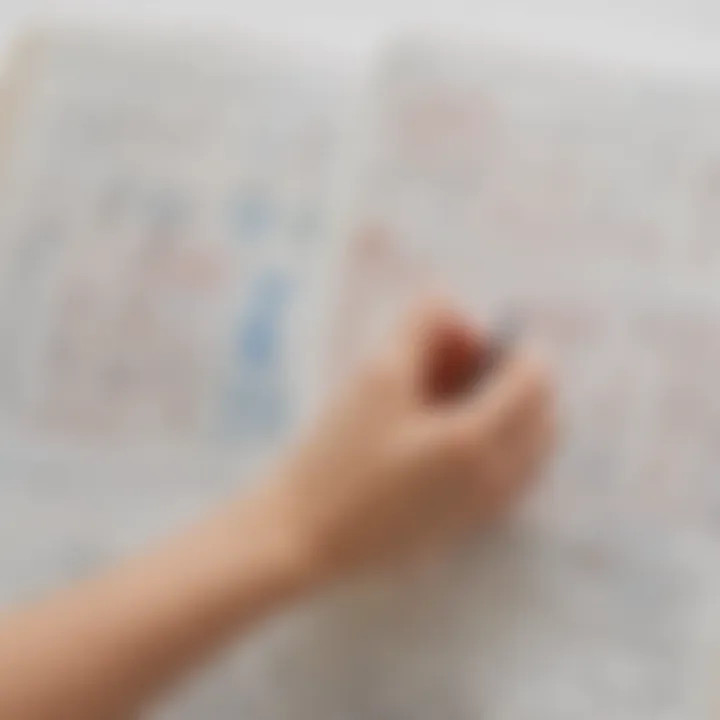
Data Quality Issues
Exploring the theme of data quality issues, we turn our attention to the critical task of addressing inaccuracies and uncertainties within datasets. Addressing issues related to data accuracy and reliability is paramount in research to uphold the credibility of findings and conclusions. By focusing on enhancing data quality, researchers can rely on the trustworthiness of their analyses to make informed decisions and draw meaningful insights. The meticulous process of identifying and resolving data quality issues ensures that research outcomes are built on a solid foundation of reliable information.
Interpretation Challenge
When confronted with the complexities of interpreting data results, researchers must navigate through the nuances of data analysis to extract meaningful information effectively. Dealing with these challenges requires a keen grasp of statistical principles and an analytical mindset to discern patterns and trends hidden within datasets. By addressing interpretation challenges head-on, researchers can unlock new perspectives and uncover valuable insights that might otherwise remain obscured. Embracing the intricacies of data interpretation is key to deriving actionable conclusions from research data and advancing scientific knowledge.
Bias and Confounding Factors
In the realm of data analysis, identifying and mitigating factors that impact the accuracy of analytical results is of utmost importance. Bias and confounding factors have the potential to skew data interpretation and lead researchers astray in their analytical pursuits. By understanding these influential elements, researchers can implement strategies to minimize their effects and ensure the objectivity of their analyses. The critical task of identifying and mitigating bias and confounding factors underscores the rigorous approach required in data analysis to uphold the integrity and validity of research findings.
Best Practices in Data Analysis
In this segment of our comprehensive guide on Analyzing Data in Research, we delve into the critical aspect of Best Practices in Data Analysis. Effective data analysis hinges on the application of best practices to ensure accuracy, reliability, and validity of the results obtained. By adhering to established methodologies and standards, researchers can enhance the quality and credibility of their research outcomes. Emphasizing the significance of meticulous data handling, proper documentation, and adherence to ethical considerations, the section sheds light on indispensable practices that contribute to robust data analysis.
Data Cleaning and Preprocessing
Ensuring data integrity through cleaning and preprocessing
Data cleaning and preprocessing play a pivotal role in maintaining data integrity and mitigating errors or inconsistencies that could compromise the analysis process. By systematically identifying and rectifying inaccuracies, missing values, outliers, and other anomalies, researchers can ensure the reliability and integrity of their datasets. This meticulous approach not only enhances the quality of analysis but also boosts the credibility of the research findings. The systematic elimination of noise and irrelevant data paves the way for more accurate and insightful analysis, enabling researchers to draw meaningful conclusions with confidence.
Documentation and Reproducibility
Importance of documenting analysis steps for reproducibility
Documentation stands as a cornerstone of reproducible research, facilitating transparency, accountability, and the validation of findings. By meticulously documenting the steps involved in data analysis, researchers enable peers to replicate the study and verify the results independently. This practice not only enhances the trustworthiness of the research but also fosters scientific collaboration and advancement. Documenting analysis steps, data transformations, and algorithm implementations ensures the traceability of decisions and enhances the robustness of the analytical process.
Validation and Sensitivity Analysis
Validating results and assessing sensitivity for robust analysis
Validation and sensitivity analysis are essential components of ensuring the accuracy, reliability, and robustness of research outcomes. By subjecting the results to rigorous validation procedures and sensitivity analyses, researchers can gauge the stability and generalizability of their findings. This process helps identify potential biases, errors, or confounding factors that could impact the interpretation of results. By validating findings through multiple methods and assessing their sensitivity to varying parameters, researchers can enhance the credibility and trustworthiness of their analysis, thereby fortifying the integrity of the research outcomes.
Conclusion
The Conclusion section of this comprehensive guide on Analyzing Data in Research plays a pivotal role in tying together the various aspects discussed throughout the article. As researchers immerse themselves in the intricate world of data analysis, the Conclusion serves as a compass, guiding them towards synthesizing the information unveiled in preceding sections. This final segment encapsulates the essence of the entire research process, emphasizing the significance of diligence, precision, and critical thinking.
One of the key elements underscored in the Conclusion of this guide is the paramount importance of drawing accurate interpretations from the analyzed data. Research endeavors hinge on the ability to decipher patterns, trends, and relationships buried within the numerical constructs. The Conclusion illuminates how researchers should approach data with a discerning eye, delving beyond superficial numbers to extract conclusive insights that can steer the course of scholarly investigations.
Furthermore, the Conclusion of this guide accentuates the iterative nature of data analysis. It elucidates on how researchers must not perceive data analysis as a linear trajectory but rather as a continuous loop of exploration, validation, and refinement. By circling back to review and reevaluate analysis outcomes, researchers can fortify the robustness of their findings and ensure the integrity of their research outputs.
An intrinsic benefit of delving into the Conclusion section lies in its capacity to instill a sense of closure and direction. As researchers navigate the complexities of data analysis, the Conclusion provides a coherent endpoint, offering a framework through which to reflect on the journey undertaken. It prompts individuals to amalgamate the various facets of data analysis studied, fostering a holistic understanding that fosters a deeper appreciation for the nuances of research methodologies.
Moreover, the Conclusion acts as a compass that directs researchers towards future avenues of exploration and inquiry. It not only consolidates the insights gleaned from the analytical process but also propels researchers towards new horizons of discovery and innovation. By outlining potential extensions, implications, and applications of the research findings, the Conclusion ignites a spark of curiosity and inspiration, motivating researchers to delve deeper into the realms of data analysis.