Unveiling the Process of Analyzing Data Through the Scientific Method
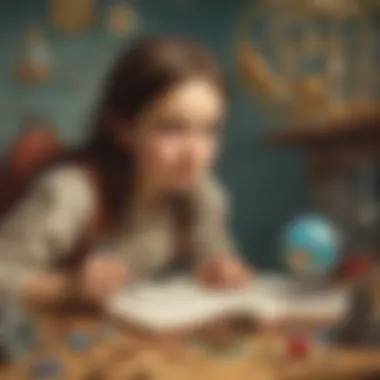
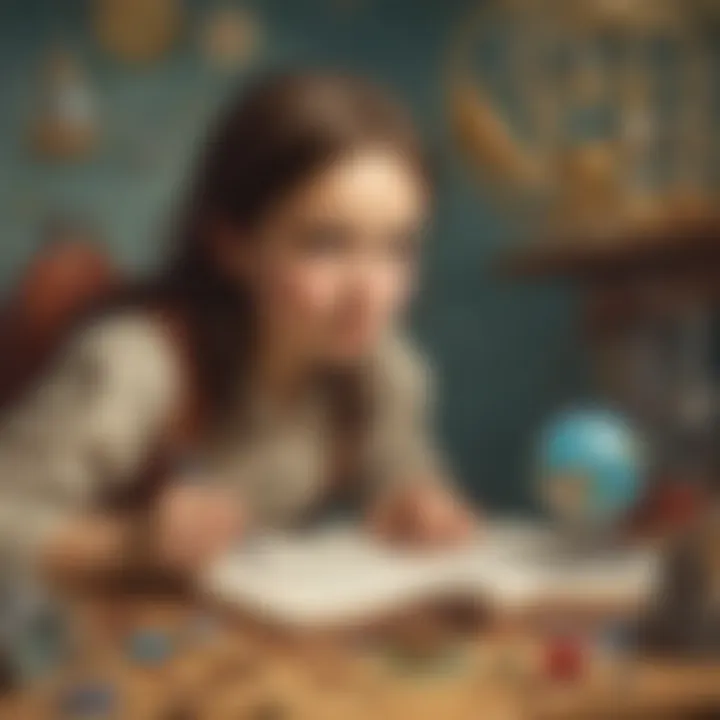
Science Fun Facts
Scientific data analysis is an enticing endeavor that unlocks the mysteries hidden within numbers and observations. Did you know that Sir Isaac Newton, a pioneer in scientific methodology, made significant contributions to calculus while studying the motion of celestial bodies? Despite his theoretical work, Newton's principles laid the foundation for modern data analysis techniques. This intriguing connection between historical figures and modern practices illuminates the evolution of data interpretation through the ages.
Discover the Wonders of Science
In the realm of data analysis, exploring various scientific concepts is akin to uncovering a treasure trove of knowledge. By delving into topics such as probability, statistical inference, and data visualization, one can appreciate the intricate tapestry that weaves together data analytics and scientific inquiry. Educational videos and animations provide engaging visuals that elucidate complex concepts, making the learning process both enriching and enjoyable for aspiring data scientists.
Science Quiz Time
Quiz time offers an interactive platform to test your understanding of data analysis principles. Multiple-choice questions challenge players to apply statistical reasoning and critical thinking skills to solve brain teasers and puzzles. By gamifying the learning experience, quizzes stimulate intellectual curiosity and foster a deeper appreciation for the systematic approach underlying the scientific method. These engaging activities not only entertain but also educate, turning data analysis into an exciting and rewarding intellectual pursuit.
Science Experiment Showcase
Embark on a journey of discovery with fun and engaging experiments that bring data analysis to life. Step-by-step instructions guide participants through practical activities that demonstrate statistical concepts in action. From creating histograms to conducting hypothesis tests, each experiment offers a hands-on opportunity to explore the nuances of data interpretation. A detailed materials list ensures that aspiring scientists have everything they need to conduct experiments safely, while accompanying safety tips and precautions prioritize a secure and informative learning environment.
Introduction to Data Analysis
Data analysis through the scientific method serves as a fundamental process of deriving insights from empirical evidence, driving decision-making and knowledge acquisition. The significance of mastering data analysis lies in its ability to provide structured approaches to interpreting information accurately. Understanding the scientific method is crucial in this context as it forms the basis of logical reasoning and evidence-based conclusions. By following systematic methods, researchers can ensure the credibility and reliability of their findings.
Understanding the Scientific Method
The concept of empirical evidence
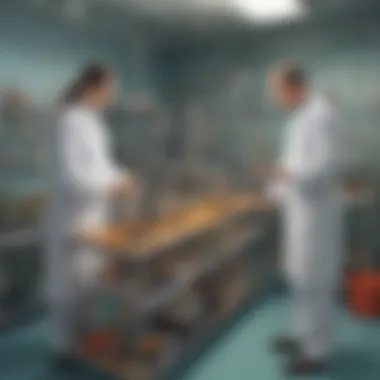
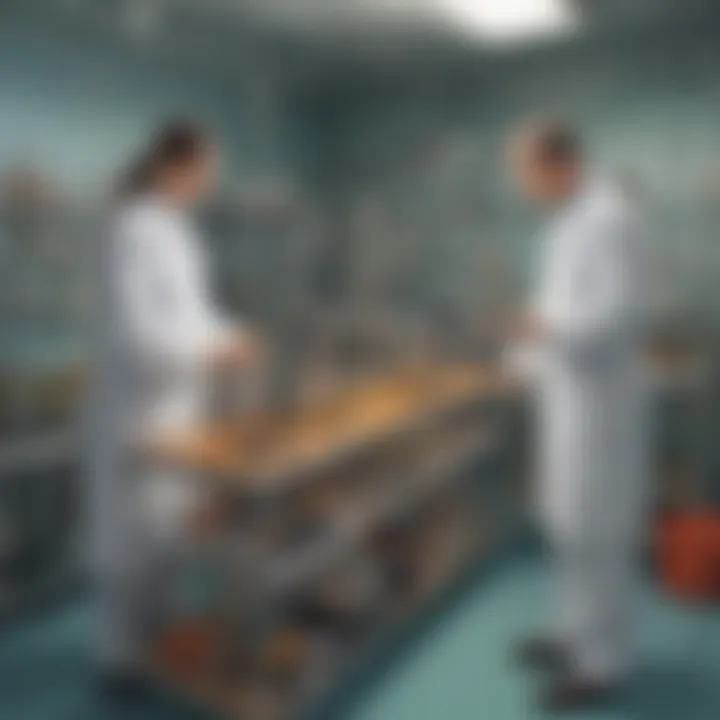
The concept of empirical evidence plays a pivotal role in data analysis. Empirical evidence refers to information acquired through observation and experimentation rather than pure conjecture or theory. Its essence lies in the ability to provide concrete, verifiable data that forms the basis of scientific inquiry. Utilizing empirical evidence ensures that analyses are grounded in reality and are not mere speculation, enhancing the validity of research outcomes. While the utilization of empirical evidence demands meticulous observation and precise measurement, its reliability and objectivity make it a cornerstone of credible data analysis.
Importance of systematic inquiry
Systematic inquiry is a foundational aspect of the scientific method. By following a structured approach to problem-solving, researchers can ensure the reproducibility and reliability of their findings. Systematic inquiry involves delineating clear objectives, defining variables, and establishing protocols for data collection and analysis. This methodical approach streamlines the research process, reducing errors and biases that may affect the outcomes. Emphasizing systematic inquiry in data analysis not only enhances the rigor of the study but also fosters transparency and accountability in research practices.
Key Steps in the Scientific Method
Observation and questioning
Observation and questioning are integral steps in the scientific method, guiding researchers in identifying patterns, anomalies, or relationships within data. Through keen observation and thoughtful questioning, researchers can unearth underlying trends or phenomena that warrant further exploration. Observations provide the empirical foundation for hypotheses, prompting researchers to formulate informed questions and hypotheses for subsequent testing. By honing observational skills and posing critical questions, researchers lay the groundwork for systematic data analysis and interpretation.
Hypothesis formulation
Hypothesis formulation drives scientific inquiry by establishing testable propositions that seek to answer specific research questions. A well-crafted hypothesis serves as a directional statement that guides the research process, outlining the expected outcomes and potential explanations for observed phenomena. By formulating hypotheses based on prior knowledge or empirical evidence, researchers can structure their experiments to validate or refute the proposed concepts. Effective hypothesis formulation fosters clear experimental design, enabling researchers to draw meaningful conclusions from their data analysis efforts.
Data Collection and Experimentation
Designing Controlled Experiments
Variables and controls
Variables and controls form the cornerstone of designing controlled experiments in scientific investigations. Variables are factors that can change or be manipulated during the experiment, while controls are kept constant to minimize outside influence on the study outcomes. The careful selection and manipulation of variables allow researchers to isolate the impact of specific factors on the observed phenomena. By controlling extraneous variables, scientists can enhance the internal validity of their findings, ensuring that any observed effects are indeed due to the manipulated variables.
Ensuring reproducibility
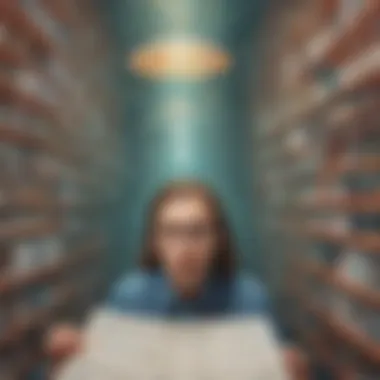
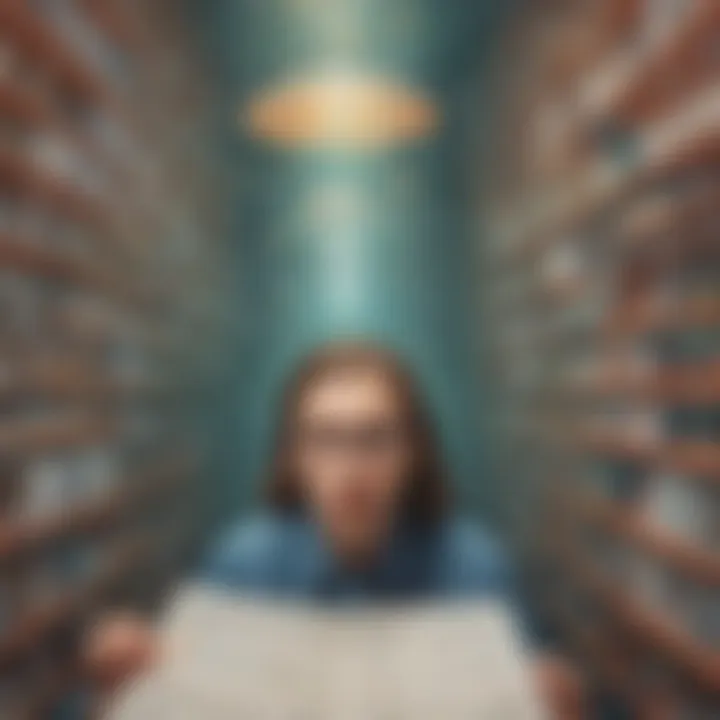
Ensuring reproducibility is a critical aspect of experimental design that emphasizes the replicability of study results. Reproducibility refers to the ability of other researchers to repeat the experiment using the same methods and materials and obtain similar outcomes. By documenting procedures meticulously and providing transparent access to data, researchers bolster the credibility of their work and facilitate the validation of findings by the scientific community. Reproducibility fosters trust in research outcomes and promotes scientific rigor and advancement.
Data Gathering Techniques
Data gathering techniques encompass the methodologies employed to collect and record information for analysis. In the realm of data analysis through the scientific method, the choice between quantitative and qualitative data plays a pivotal role in shaping the research approach. Quantitative data involve numerical information that can be quantified and statistically analyzed, providing structured insights into patterns and relationships within the data. On the other hand, qualitative data entail non-numerical information, such as narratives or observations, offering rich contextual understanding and in-depth insights into complex phenomena.
Tools and instruments
Tools and instruments utilized in data gathering serve as essential facilitators of the research process, enabling accurate data collection and measurement. These instruments range from traditional survey tools to sophisticated technological devices, each tailored to capture specific data types accurately and efficiently. The selection of appropriate tools and instruments hinges on the research objectives, data complexity, and feasibility considerations. Utilizing advanced tools can enhance data accuracy and reproducibility, while improper instrument selection may introduce biases or errors into the analysis, compromising the research outcomes.
Statistical Analysis and Interpretation
In the realm of data analysis through the scientific method, statistical analysis and interpretation play a pivotal role in deriving meaningful insights π. One of the critical elements that underscores the significance of statistical analysis is its ability to condense complex data into comprehensible summaries, aiding in the identification of patterns and trends. By employing statistical methods, researchers can quantify uncertainties and assess the variability within datasets, fostering a more robust understanding of the information at hand. Moreover, statistical analysis serves as a key instrument in hypothesis testing, guiding researchers in making informed decisions based on concrete evidence and rigorous empirical observations.
Utilizing Statistical Methods
Descriptive vs. inferential statistics
Delving into the dichotomy between descriptive and inferential statistics provides a lens through which researchers can both summarize data patterns and draw inferences about broader populations. Descriptive statistics focus on detailing the characteristics of a dataset, offering insights into central tendencies, dispersion, and graphical representations. In contrast, inferential statistics extend beyond mere description, allowing researchers to make generalizations and predictions based on sample data. The advantageous nature of descriptive statistics lies in its ability to simplify complex information into meaningful summaries, enabling researchers to communicate findings effectively. Conversely, inferential statistics offer the advantage of drawing conclusions that extend beyond the observed data, providing a broader understanding of the underlying relationships and patterns within a given study.
Hypothesis testing
A cornerstone of the scientific method, hypothesis testing holds immense value in the process of data analysis π§ͺ. By formulating clear hypotheses and subjecting them to rigorous testing, researchers can ascertain the validity of their assumptions and draw reasoned conclusions based on empirical evidence. The key characteristic of hypothesis testing resides in its structured approach to evaluating conjectures through statistical analysis, thereby fostering a methodical and replicable investigative process. This method proves beneficial in identifying causal relationships and verifying the accuracy of proposed explanations, enhancing the credibility and robustness of research outcomes. While hypothesis testing offers the advantage of providing concrete answers to research questions, it can also pose challenges in cases where design flaws or biases distort the interpretation of results.
Drawing Conclusions
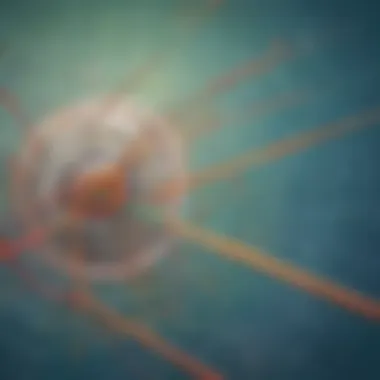
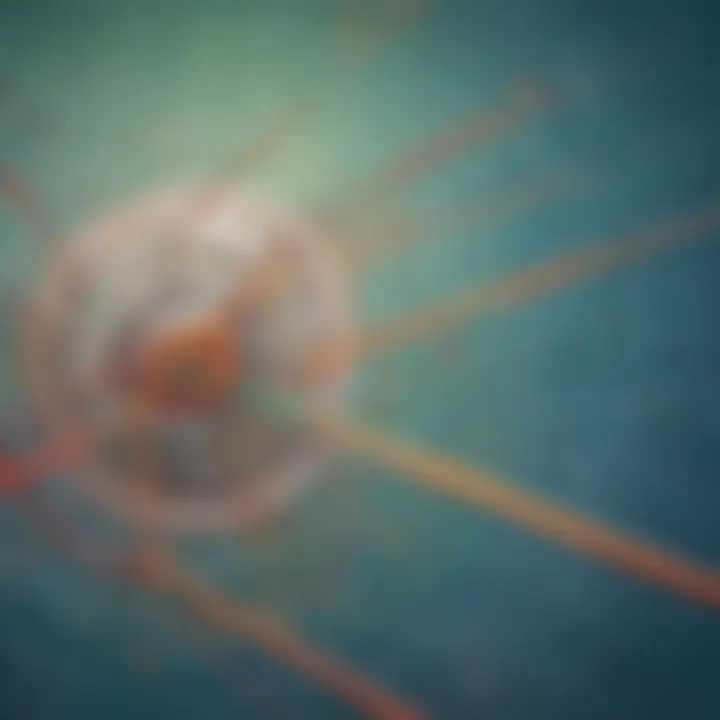
Interpreting data trends
The task of interpreting data trends serves as a mechanism through which researchers can unravel the underlying patterns and relationships embedded within datasets π. This analytical process involves scrutinizing variations in data points over time and discerning the implications of these fluctuations on the research findings. A key characteristic of interpreting data trends lies in its ability to reveal the directional movement of variables and elucidate the factors influencing their trajectory. This practice proves beneficial by granting researchers a nuanced understanding of how variables interact and evolve, facilitating informed decision-making and hypothesis refinement. Despite its advantages, interpreting data trends may present challenges in scenarios where the data is noisy or exhibits irregular patterns, warranting careful analysis and consideration of alternative explanations.
Identifying correlations
The ability to identify correlations within datasets stands as a fundamental aspect of data analysis, shedding light on the relationships between variables and guiding subsequent research inquiries π. Highlighting the strength and direction of associations between different data points, correlation analysis aids researchers in discerning patterns and predicting future outcomes. A key characteristic of identifying correlations is its capacity to quantify the degree of relationship between variables, offering insights into how changes in one variable influence another. This feature proves advantageous in uncovering hidden dependencies and guiding the formulation of hypotheses grounded in empirical observations. Despite its benefits, identifying correlations may pose challenges in cases where spurious relationships or confounding variables obscure the true nature of associations, necessitating robust validation techniques and careful scrutiny.
Ensuring Data Integrity
In the vast realm of data analysis, the topic of Ensuring Data Integrity stands as a pivotal element that can not be underestimated. Data Integrity is the bedrock of any successful analytical endeavor, serving as the guardian of accuracy and reliability in the sea of information. Without Ensuring Data Integrity, the entire process of analysis falls into jeopardy, risking faulty conclusions and misguided insights. Therefore, within the context of this article, the emphasis on Ensuring Data Integrity is paramount, elucidating the significance of maintaining the purity and consistency of data throughout the analytical journey.##
Validity and Reliability
Evaluating research methodologies
When delving deep into the intricacies of Validity and Reliability, one encounters the critical facet of Evaluating research methodologies. This particular aspect plays a substantial role in fortifying the foundation of data analysis, ensuring that the chosen methodologies are robust and capable of yielding meaningful results. Evaluating research methodologies involves a meticulous assessment of the processes, techniques, and approaches employed during the data collection and analysis phases. The key characteristic of Evaluating research methodologies lies in its ability to validate the credibility and effectiveness of the chosen research framework. It stands as a reliable mechanism that safeguards the integrity of the analysis by scrutinizing the methodologies for any inherent biases or shortcomings. Despite its meticulous nature, Evaluating research methodologies proves to be a beneficial choice for this article as it guarantees a systematic and rigorous evaluation of the research procedures, contributing to the overall credibility and trustworthiness of the analytical outcomes. Its unique feature lies in its capability to enhance the transparency and replicability of the research, ensuring that the findings can withstand critical scrutiny and external validation. However, like any methodological approach, Evaluating research methodologies is not devoid of disadvantages, as it can be time-consuming and resource-intensive, requiring a comprehensive understanding of research design principles and analytical techniques.
Minimizing biases
In the intricate web of Validity and Reliability, the imperative role of Minimizing biases emerges as a key determinant of analytical authenticity. Minimizing biases involves a strategic process of identifying, acknowledging, and mitigating any potential factors or influences that could skew the results or distort the conclusions of the analysis. The key characteristic of Minimizing biases lies in its preventive nature, aiming to weed out any preconceived notions, personal inclinations, or external pressures that might taint the objectivity of the analytical findings. This approach is a popular choice for this article as it reinforces the fundamental principle of unbiased data interpretation, fostering a transparent and impartial analytical environment. The unique feature of Minimizing biases is its capacity to enhance the reliability and validity of the analysis by promoting a neutral and objective stance towards the data, thereby minimizing the risk of erroneous conclusions or misleading interpretations. Nevertheless, Minimizing biases comes with its own set of challenges, including the complexity of identifying subtle biases, the subjectivity in bias assessment, and the constant vigilance required to maintain a bias-free analytical process.
Ethical Considerations
Respecting data privacy
Within the realm of Ethical Considerations, the facet of Respecting data privacy emerges as a fundamental cornerstone of data analysis ethics. Respecting data privacy entails upholding the confidentiality, anonymity, and security of the data sources and ensuring that the personal information or sensitive data is safeguarded from unauthorized access or misuse. The key characteristic of Respecting data privacy lies in its commitment to protecting the rights and privacy of individuals whose data is being analyzed, fostering a culture of trust and respect in the data analysis process. This aspect is a beneficial choice for this article as it underscores the ethical responsibility of data analysts to handle information ethically and responsibly, aligning with data protection regulations and privacy standards. The unique feature of Respecting data privacy is its potential to enhance data credibility and reliability by assuring data providers that their information is handled with integrity and confidentiality, fostering greater cooperation and data sharing. However, Respecting data privacy also poses challenges, including the need for robust data security measures, compliance with data protection laws, and the constant vigilance required to prevent data breaches or unauthorized disclosures.
Avoiding conflicting interests
Complementing the ethical framework of Ethical Considerations is the pivotal aspect of Avoiding conflicting interests, which plays a crucial role in maintaining the integrity and impartiality of the data analysis process. Avoiding conflicting interests involves identifying, disclosing, and mitigating any personal, professional, or financial conflicts that could potentially compromise the objectivity and integrity of the analysis. The key characteristic of Avoiding conflicting interests lies in its emphasis on transparency, accountability, and independence in data analysis, ensuring that the analytical process remains free from undue influences or biases. This aspect is a popular choice for this article as it aligns with the ethical standards and best practices governing research and data analysis, emphasizing the importance of integrity and honesty in disclosing potential conflicts of interest. The unique feature of Avoiding conflicting interests is its capacity to bolster the credibility and trustworthiness of the analysis by demonstrating a commitment to ethical conduct and unbiased data interpretation. Nonetheless, Avoiding conflicting interests presents its own set of challenges, including the complexity of identifying subtle conflicts of interest, the ethical dilemma of balancing competing interests, and the need for clear guidelines and protocols to address conflicts in data analysis.